The Massive AI Scalability Revolution That Is Coming for Us
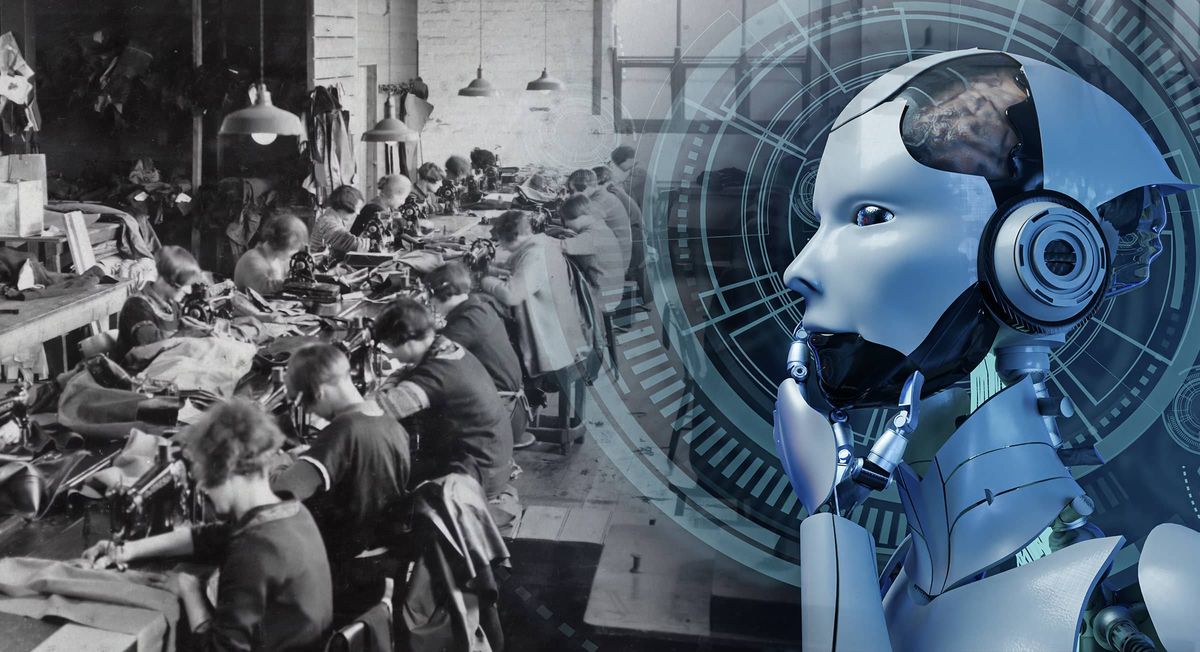
The dream of making it big by starting a startup has captured the imaginations of many aspiring entrepreneurs. Yet, the harsh reality is that many such dreams falter and fade. At the other end of the spectrum, a number of small and medium-sized businesses (SMBs) have achieved great success without ever being touched by the startup hype. Often starting from humble beginnings as solo initiatives to solve customer problems, these companies have grown over time into substantial enterprises - an evolution well exemplified by the "German Mittelstand".
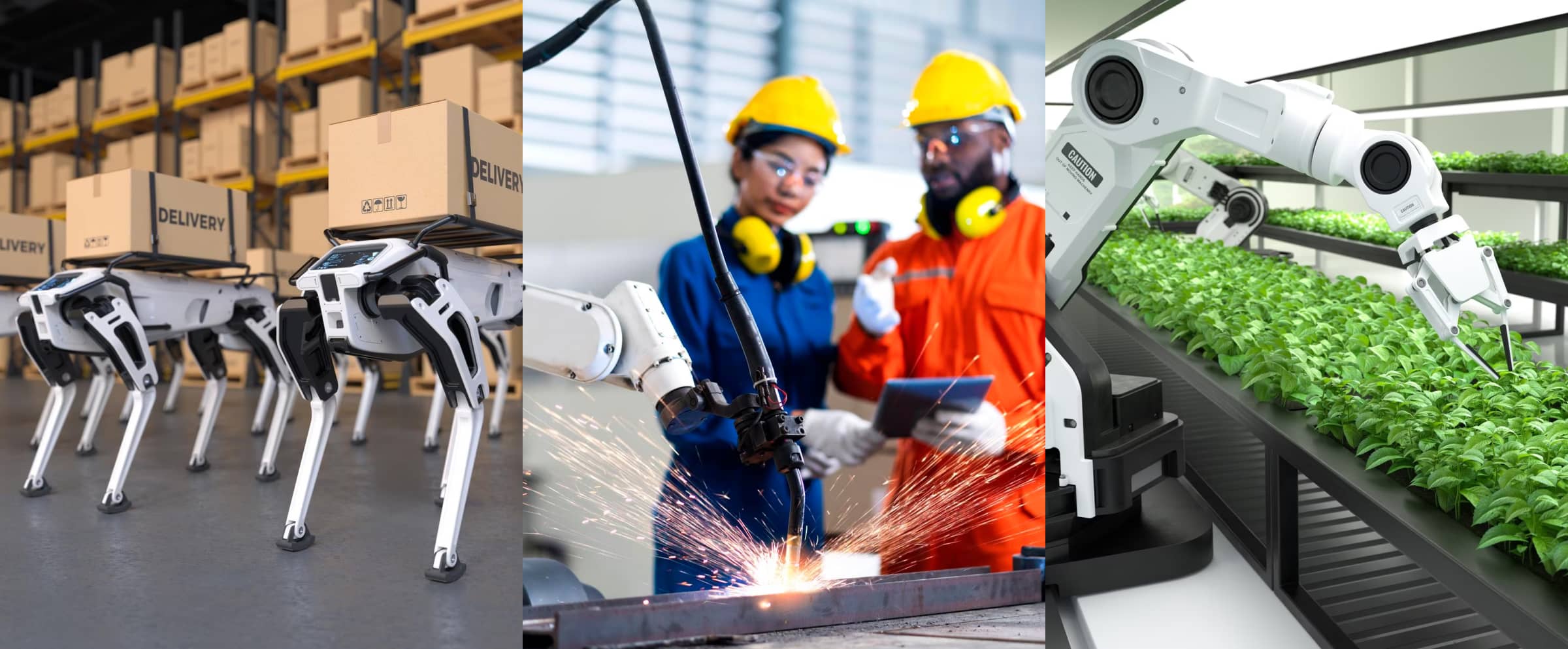
However, an Achilles' heel often remains: the reliance on human labor to scale, and the persistent and sometimes even stubborn commitment to depending on basic tools such as Excel, Word, and email to deal with ever-increasing amounts of data and complex processes. Relying on these simple tools far beyond the use case for which they were originally designed introduces inefficiencies that not only limit a company's ability to scale, but actually create more problems as the company tries to grow.
This article is about to take you on a journey of a company that has traveled this very road. From humble beginnings to hitting a wall with a team of 50 and $10M in revenue. However, by harnessing the power of the proper Data Engineering tools and Machine Learning models, they were able to overcome the limitations that once held them back. So buckle up as we plunge into a future where the "unscalable" is redefined and made scalable, powered by the full potential of AI and Machine Learning. Be prepared to be inspired, educated, and intrigued as we explore this exciting new frontier.
The Limitations of Traditional Algorithms
Let's dive into the fascinating journey of AmazingX - the pseudonym we're using for our client, whose identity we must keep confidential. As a thriving business on Amazon, AmazingX sells around 600 distinct products, generating an impressive $10M in revenue annually, and is maintained by a diligent 50-person team. However, e-commerce on Amazon is a whole different ball game, significantly diverging from traditional commerce's long-term focus on a few products in a distinctive niche. It leans heavily on the unpredictable patterns of short-term demand and is increasingly governed by fleeting hype cycles.
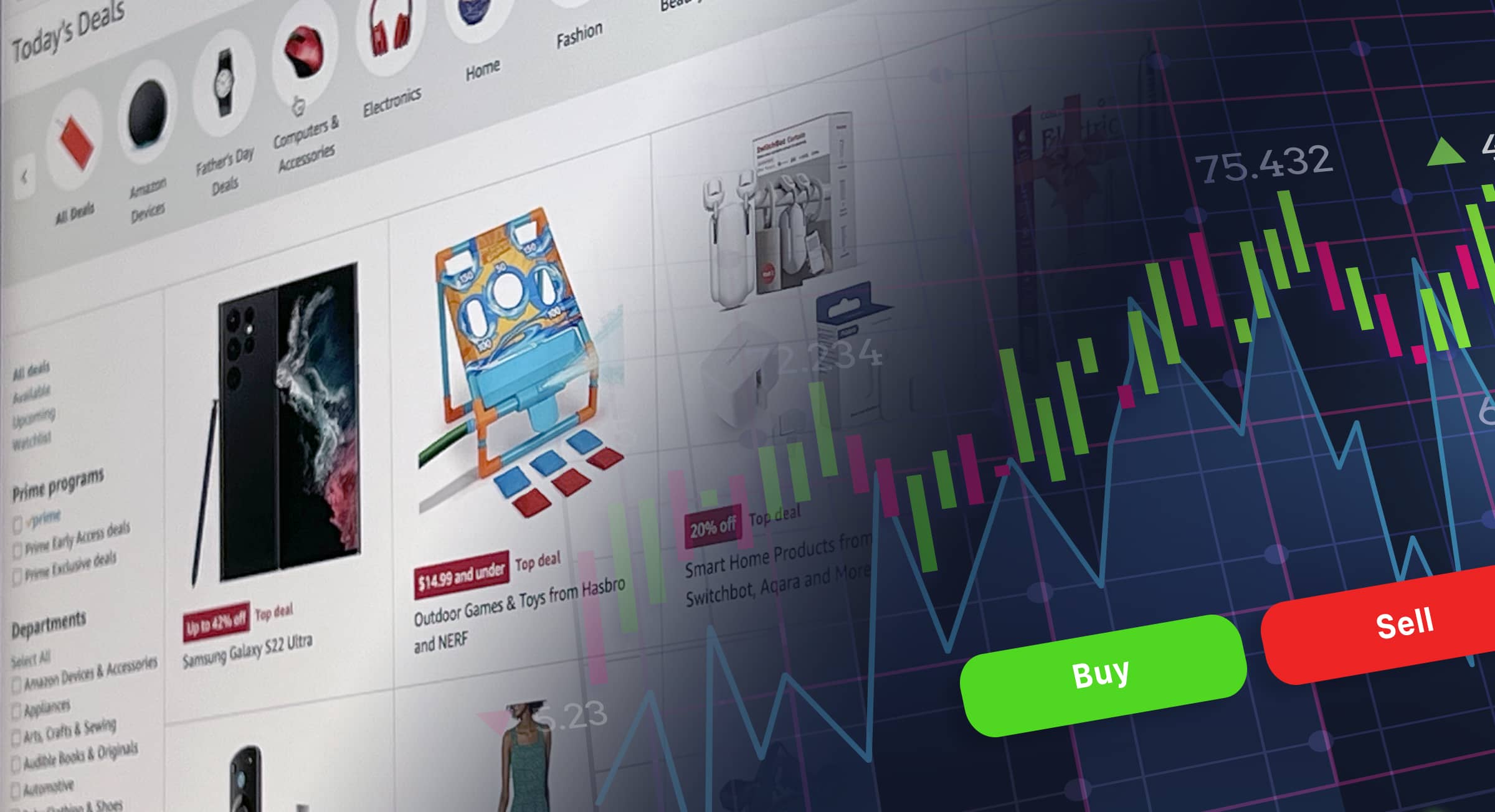
To navigate this space, you don't stockpile large quantities of items hoping for sales spread out over years. Instead, the strategy is to buy smaller, yet substantial, amounts of stock and aim for sales within days or weeks. Thus, the primary measure of success shifts from overall sales volume to sales velocity - that is, sales per product per day.
The journey of AmazingX has been nothing short of remarkable, blossoming from a one-person enterprise into a 50-strong operation in just a few years. The heartbeat of the business lies in the product research department, which tirelessly scouts for the latest trends and makes informed stock purchasing decisions. However, even with a sizable team, certain limitations exist.
Humans, no matter how proficient, are limited in their capacity to analyze vast volumes of data. This is where tools like Excel step in, providing a helping hand to humans by automating certain tasks like data sourcing (via web scraping) and converting strategic ideas into actionable algorithms. However, as is the case with natural growth, complexity increased to a point where the algorithm became too convoluted for anyone to fully grasp. Coupled with the inherent error rate of using Excel sheets and humans for processing large amounts of data, this led to a widespread lack of trust in the company's data, algorithms, and processes. Consequently, everything had to be double-checked and verified before any purchase decision could be taken, causing enormous inefficiencies and dissatisfaction within the team.
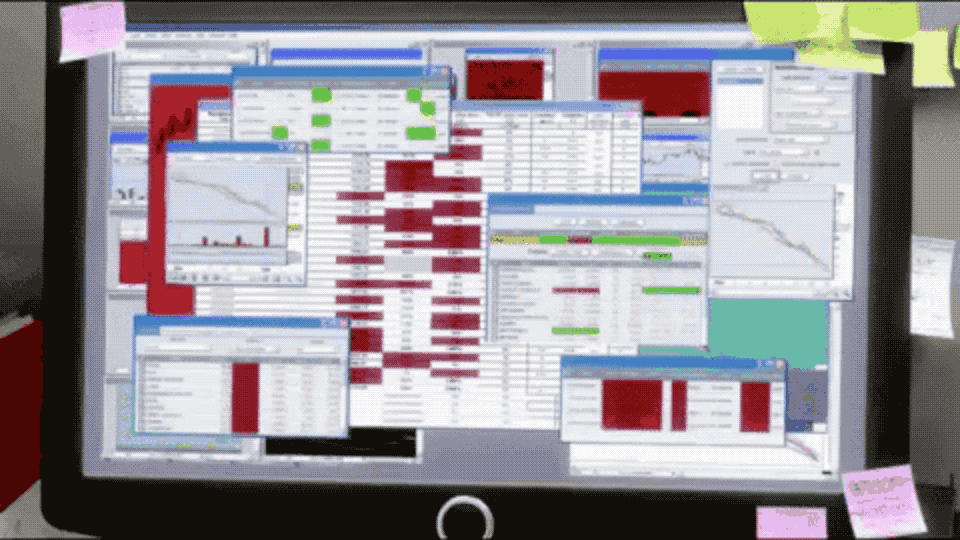
The business found itself gridlocked at its current size, unable to scale further despite a plethora of opportunities. It got to a point where employees had to monitor their processes even during holidays due to the complex, fragile, and interdependent nature of the systems. In essence, the entire company was at risk if one thing went wrong. A clear indication that it was time for a change.
Harnessing the Power of Data Engineering & Machine Learning
That's where we, SIROC, were brought in. We were tasked with assessing the situation and providing a solution that could break through AmazingX's current limitations.
We identified three main challenges and suggested the following technical solutions for each:
Step 1: Democratizing Data
Imagine, all of AmazingX's knowledge was locked away in hundreds, maybe even thousands, of Excel sheets, each one uniquely designed by different employees. Figuring out if the data was still accurate or if the linked processes were effective was virtually impossible. Our first task was to free all this locked-up data from Excel. We set up data pipelines to funnel this information into proper data lakes and data warehouses. Then we needed to calculate the error of the current algorithm to establish a baseline and a reference for what comes next.
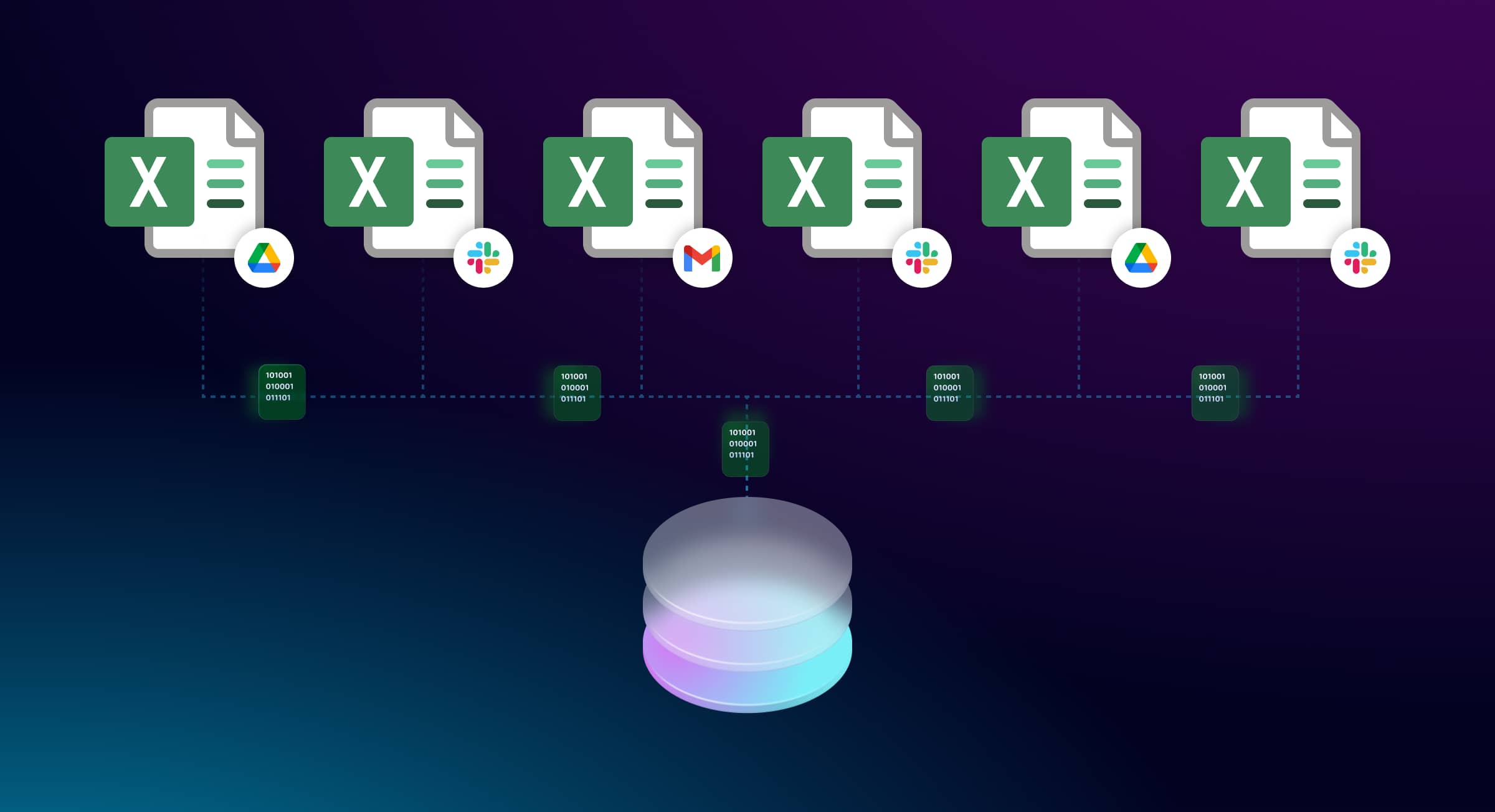
Step 2: Defining Input Features
With so much data available, how do you decide which data points (or features) are really useful? The reality is that when we start building new machine learning models, we may have some ideas, but we can't be fully sure. But we have to start somewhere. Based on the team's experience running the business to date, we worked with them to select the following features as a starting point:
- Price: Both buy and sell price are the most obvious factors that impact our sales velocity.
- Time: Weekdays vs. weekends, daytime vs. evening
- Seasonality: Summer vs. Winter
- Special events: e.g. Christmas, Mother's Day, etc.
But we didn't stop there. We continued to take other factors like consumer behavior, social media trends, and even weather forecasts into account to further improve our model.
Step 3: Choosing the Right ML Model Architecture
There are so many ML architectures to choose from, such as linear regression, logistic regression, neural networks, to name a few. And it's hard to know in advance which one will produce the best results. That's where our expertise becomes invaluable. Years of experience allow us to choose the right model and parameters to start with.
Step 4: Training and Integrating the Model
Then we begin training the model, continually feeding it more data while experimenting with different features and Machine Learning models to achieve the level of accuracy we need.
Once we have a model we're happy with, we start replacing the old algorithm with our new model in the existing IT system and processes. This is the integration phase.
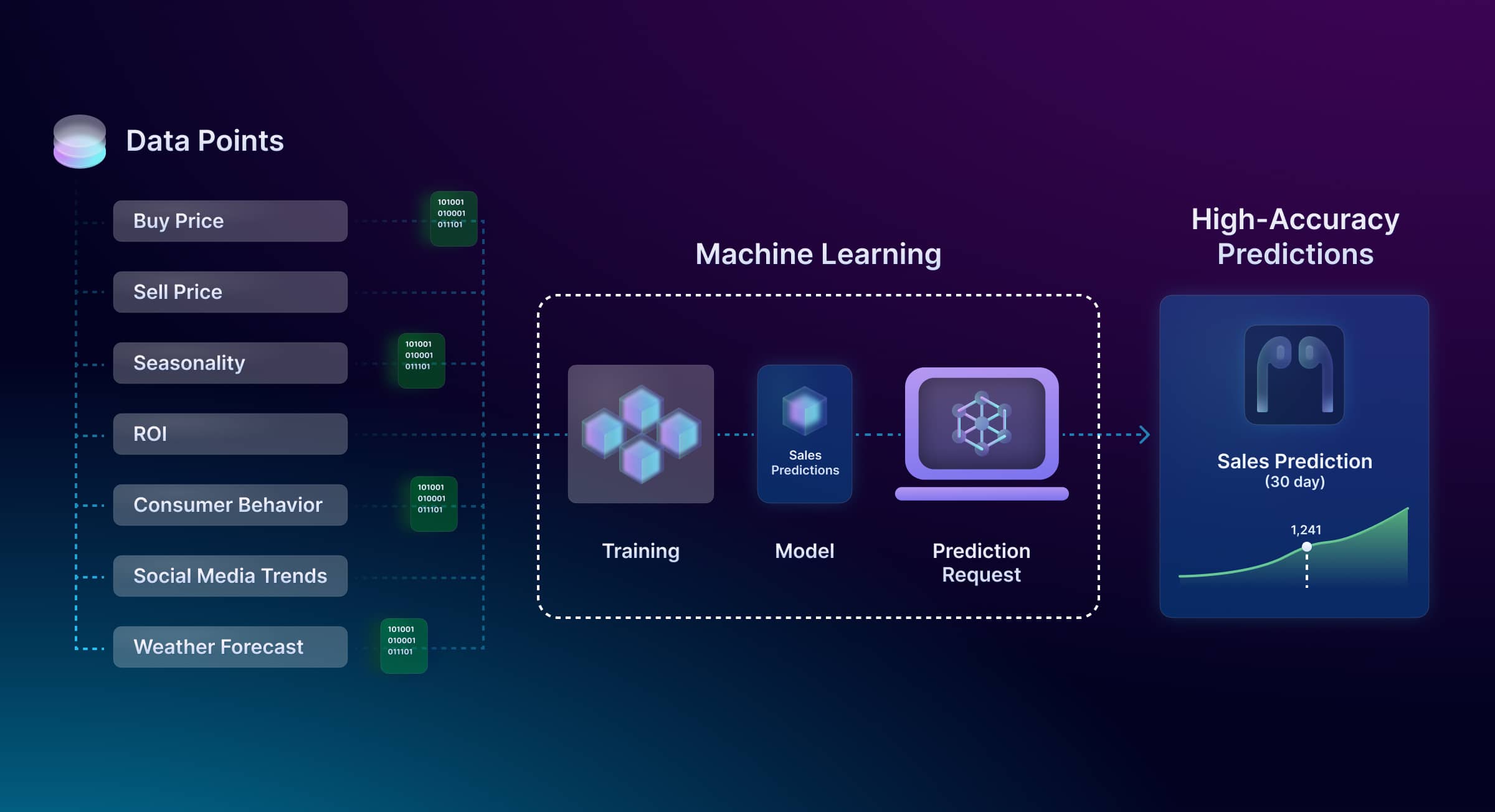
Step 5: Monitoring
Furthermore, we always establish a system monitoring process. We set daily Key Performance Indicators (KPIs) to ensure that our model is always on target. In this specific project, we aim to maintain a 95% accuracy by continually comparing our predicted sales velocity with the actual sales velocity.
If accuracy drops significantly over time, we reassess the situation, look at new data, and retrain the model with any changes that might have occurred, like shifts in consumer behavior or new trends.
Outcome & Benefits
"We were able to increase the number of daily purchase suggestions from 600 to an astounding 8000 - a 13-fold increase."
Data & IT Infrastructure Overhaul
We were able to fix a disorganized data and IT infrastructure that had been managed by far too many people. We replaced an unreliable system that was prone to constant disruptions when people were on vacation or otherwise unavailable.
With our new Data Engineering, Automation and Monitoring systems, all of these people have been freed from the day-to-day stress of keeping the business running and can now focus on more creative tasks, thinking about how to improve the actual business.
Vertical Scalability Unleashed
In the old system, product purchase suggestions were manually researched and verified by people. Adding more products to the catalog meant hiring more people.
Now, pretty much all of that is fully automated, and instead of spending 5 minutes of employee time per suggestion, it takes 30 seconds just to have a human check that what our models are spitting out is not completely wrong.
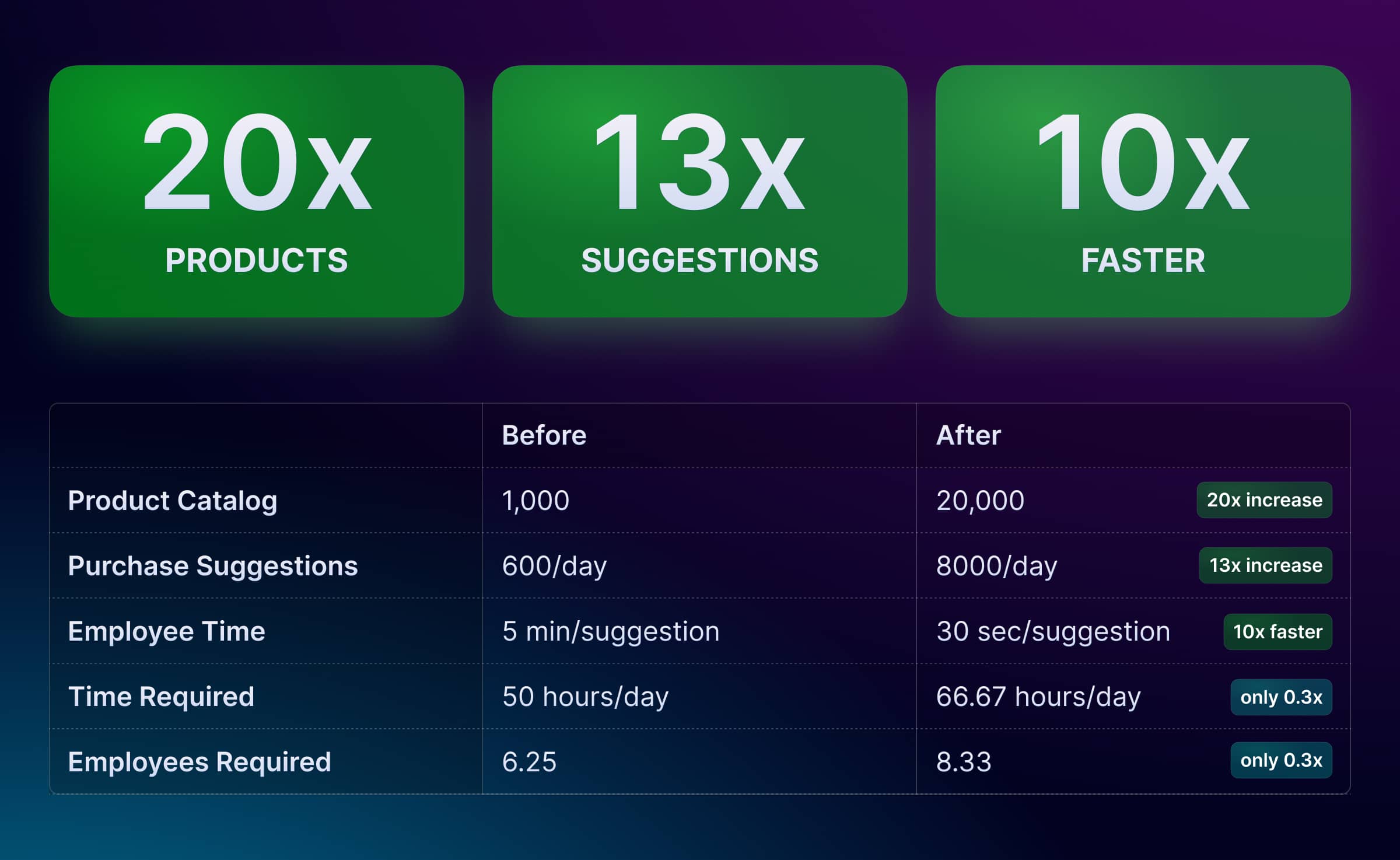
So what does that mean? With a staff increase of only 33%, we were able to amplify the daily purchase suggestions from 600 to an astounding 8000—a 13-fold increase. This also expands the potential product catalog to 20,000 items, up from a mere 1000.
Capital Efficiency Boost
We didn't stop at just automating the old processes. With our model now capable of processing thousands of purchase proposals per day, we further refined the model to prioritize purchase options with a higher return on investment (ROI). By empowering the business to continually reassess capital allocation, we were able to increase the overall ROI from 35% to a remarkable 95%.
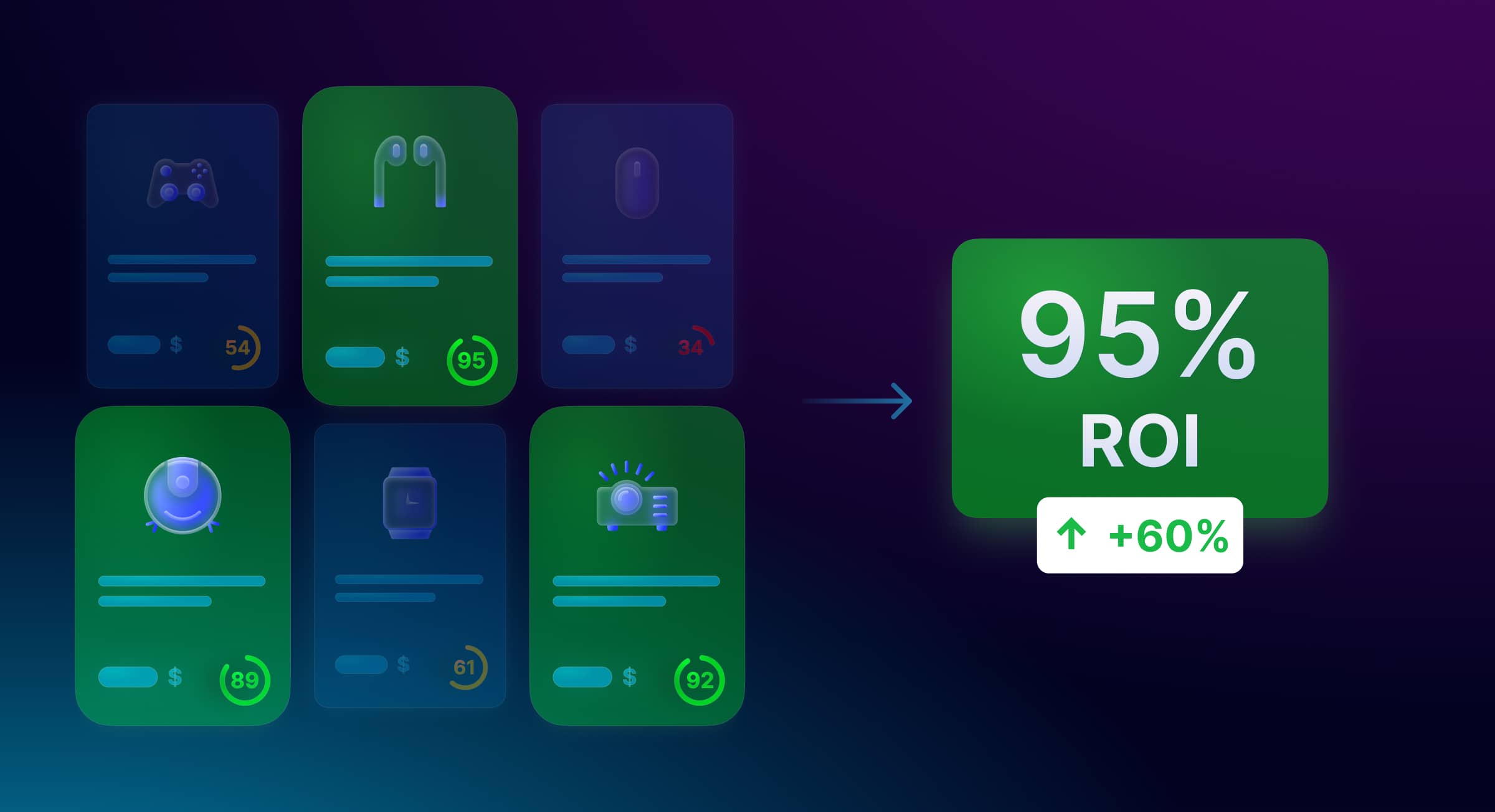
Conclusion
While a considerable number of businesses have begun to automate their operations, many still rely on outdated tools like Excel, and heavily depend on their staff to manually carry out tasks within their automation chain. Not only is this prone to error, but it also limits your ability to scale your business.
In the midst of the current buzz around Generative AI, many are overlooking the significant gains that can be achieved through proper Data Engineering combined with reliable and time-tested Machine Learning models.
There is a wealth of opportunities in the market, with many 'low hanging fruits' available for businesses willing to modernize and elevate their operations to the next level.
About SIROC
We hope this case study has sparked your interest in how Data Engineering and Machine Learning can be applied to any business to achieve new heights of scalability and profitability.
If you need assistance or advice with data analytics, data engineering, or integrating ML/DL models into your business, don't hesitate to reach out to us at SIROC. We specialize in developing custom AI solutions tailored to your needs, such as forecasting models, recommender and decision maker models, as well as anomaly detection models.
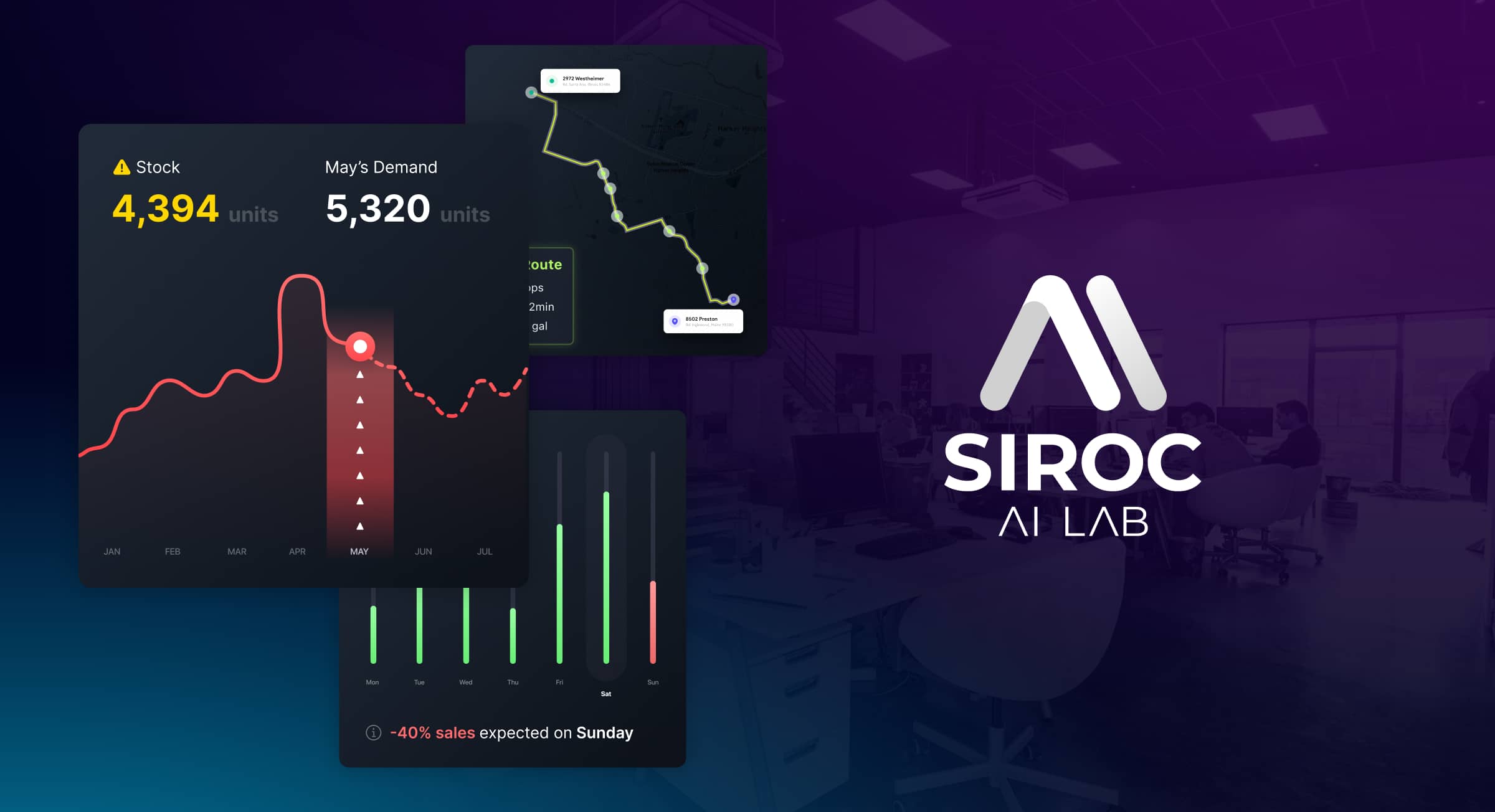
Not only do we have an extensive collection of pre-trained in-house models, but we also collaborate with industry-leading partners such as OpenAI and Aflorithmic to deliver cutting-edge solutions to optimize and automate your business. Find out more about our products and solutions on our website.
Images provided by Freepik.